Quality assurance is our top priority
I started Flashcard Space because I couldn’t find Spanish flashcards that would meet the quality bar I wanted when learning. At first glance, a flashcard might seem an easy thing to design. However, there are quite a few aspects to consider to make it a valuable and reliable learning aid.
The decks I usually find on Quizlet or Anki deck repository have problems with one or more of the following aspects:
- typos (I encountered them much more frequently than I expected)
- inaccurate translations
- lack of audio or poor-quality audio
- lack of word usage in context
Here’s an example of a contrast between a hastily designed flashcard, quite typical for Quizlet and other student-created resources, and a more refined one:
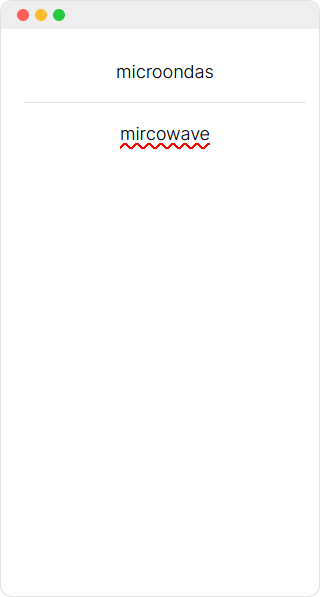
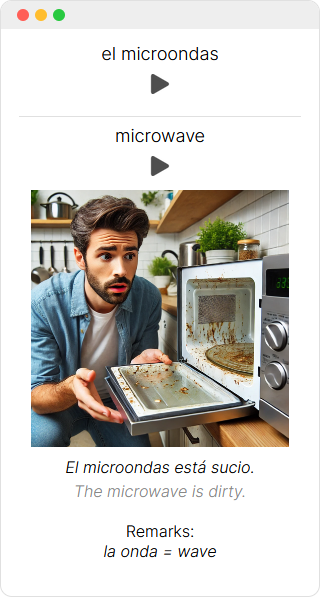
The Flashcard Space project aims to deliver flashcards of the second kind, allowing us to supplement your language learning with efficient resources to build up your vocabulary.
Our Quality Assurance process
As I write, in 2024, more content found online is being generated by Generative AI tools like ChatGPT, with creators sometimes taking shortcuts that they shouldn’t. Here’s where we stand on that matter.
In Flashcard Space, we’ve developed our authoring tools to help us create quality flashcards. Those tools proudly use a few state-of-the-art AI models to help design and draft flashcard sets. It greatly reduces the burden of repetitive work and helps make this project economically viable. However, quality assurance is always left to a human expert who corrects the mistakes (and might use alternative sources like dictionaries, online forums, and their own experience).
We believe that modern AI models are good at working with languages and help save time, but they aren’t yet good enough to fully automate the process and achieve an acceptably low rate of mistakes.
Here’s how our tooling looks. You might see that author has to manually resolve plenty of potential warnings in flashcard drafts before a flashcard gets approved to the final product:
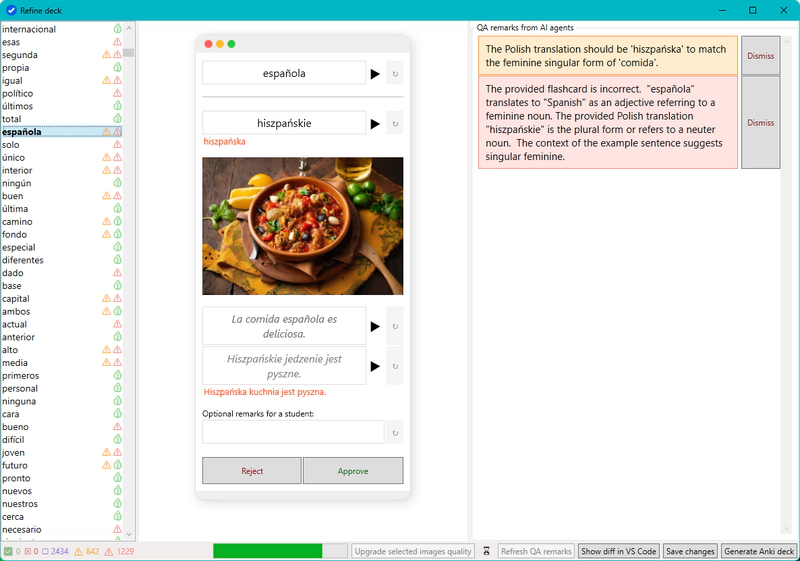
AI models used in the process
For transparency, here’s a list of AI models currently used in our process:
- OpenAI’s
gpt-4o
is used to classify words into parts of speech (nouns, adjectives, verbs, …), to generate sentence examples, and perform first pass of the validation - Google’s
gemini-1.5-pro-002
is used for a second pass of validation to bring up the potential issues to author’s attention. It uses a different AI model and prompt thangpt-4o
, often leading to additional insights. - Anthropic’s
claude-3-5-sonnet-20241022
is used for a third pass of validation to bring up the potential issues to author’s attention. - The
stable-diffusion-xl-base-1.0
engine is used to generate image candidates for each flashcard. Then the final image is selected by a human (or all are rejected). - The
flux.1-dev
model is used to generate illustrations in our newer flashcard sets.
We generally stick to the most recent models in their most premium variant (except for the image generation model where we took the more conservative approach to avoid licensing issues).